In-Database AI Agents: Teaching Claude to Use Tools With Pgai
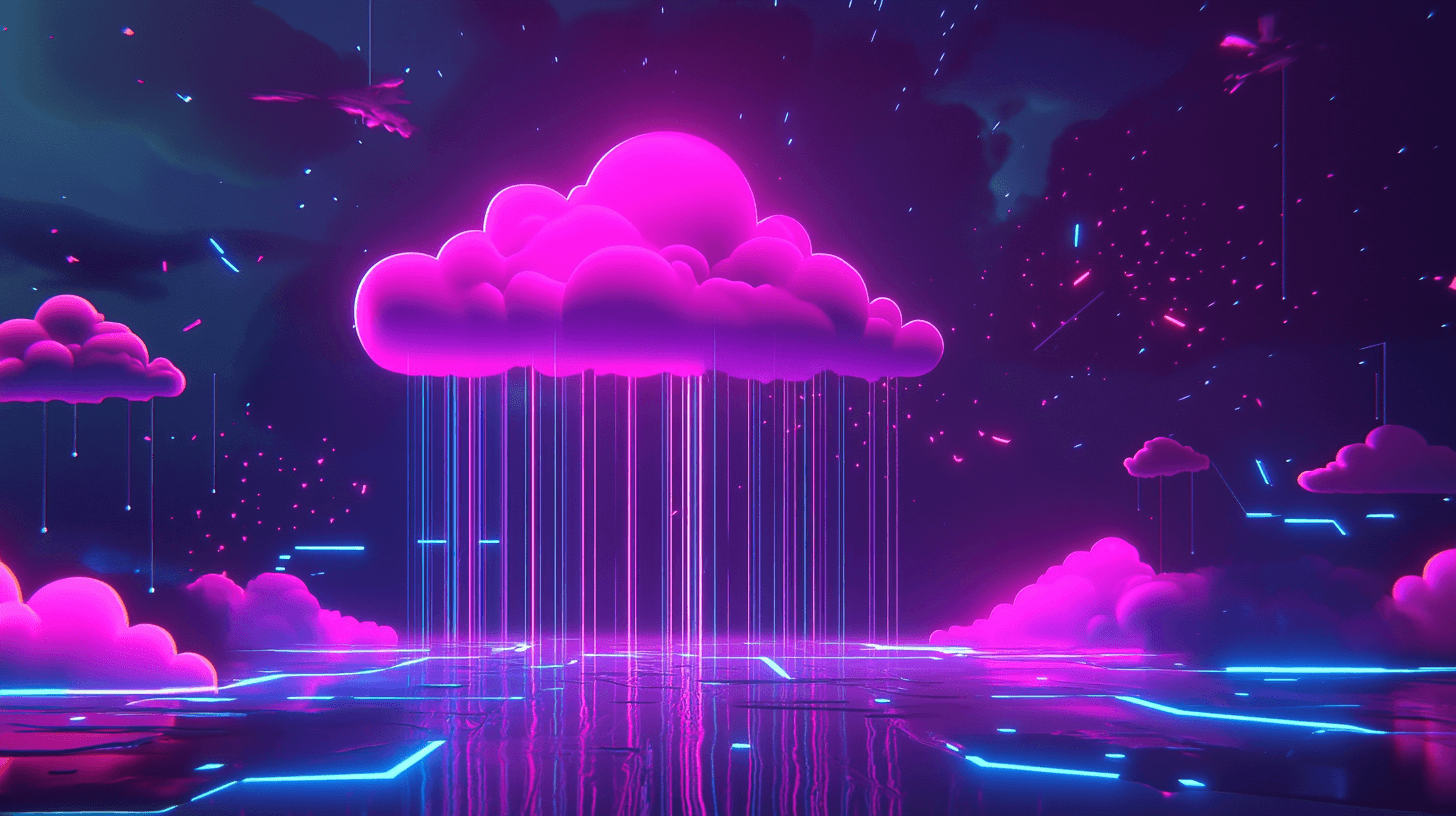
Imagine asking your device to order groceries or book a flight, and it seamlessly takes care of everything—no apps to open, no extra steps. It’s down to AI agents—computer programs designed to interact with their environment by perceiving data, interpreting it through algorithms, and acting on it to achieve specific goals. Before large language models (LLMs), AI agents primarily operated on rule-based systems, where predefined instructions and logic determined their actions.
With LLMs capable of understanding language, we can combine them with execution chains to perform specific actions. The Claude family of LLMs, for example, is equipped to use external tools and APIs, making it easier to develop custom AI agents. In this article, we will show you how to create AI agents within PostgreSQL’s database to reduce latency and simplify your architecture with the help of the pgai PostgreSQL extension.
AI Agents, Claude, and Pgai
Let’s start by understanding AI agents and discussing the components required to make one.
Understanding AI agents
An AI agent is a program that interacts with its environment to achieve human-defined objectives. LLM agents, specifically, utilize a LLM for reasoning and APIs for execution. Here's a breakdown of the key components of an LLM-powered agent:

- The agent core is the decision-making module that manages agent logic, including goals, tool execution, planning guidance, and memory coordination.
- The memory module stores the agent's previous actions and interactions, with short-term memory tracking immediate tasks and long-term memory storing broader interactions.
- The tools are the third-party APIs that agents use to perform tasks in the real world.
- The planning module decomposes complex problems into smaller tasks and refines execution plans, often using techniques like ReAct to improve reasoning and responses.
Agents can be classified based on various criteria. Here's a breakdown of these classifications:
- Reactivity vs. proactivity:
- Reactive agents:
- Respond to immediate stimuli from their environment
- Take actions based on real-time inputs without long-term planning
- Proactive agents:
- Take the initiative and plan ahead to achieve specific goals
- Consider future states and outcomes, not just immediate stimuli
- Reactive agents:
- Environment:
- Fixed environment:
- Operates within a static set of rules that do not change
- The agent's interactions are predictable and consistent
- Dynamic environment:
- Operates in an environment that is constantly changing
- Requires agents to adapt to new situations and unpredictable changes
- Fixed environment:
- Single vs. multi-agent systems:
- Single-agent system:
- Involves one agent operating independently to achieve its goals
- Multi-agent system:
- Involves multiple agents working together to achieve a common goal
- Agents must coordinate actions and communicate to accomplish objectives
- Single-agent system:
AI agents serve many benefits in various industries:
- Finance: They optimize trading, risk assessment, and fraud detection. AI agents like robo-advisors (e.g., Betterment, Wealthfront) provide personalized investment advice by analyzing market trends and customer portfolios.
- Power industry: AI agents enhance energy distribution and demand prediction. AI-driven systems like GE's Predix predict energy demand and optimize grid operations.
- Transportation: They help improve traffic management and logistics. AI agents in logistics, such as those used by UPS, optimize delivery routes by analyzing real-time traffic data, cutting down fuel costs and delivery times.
- Healthcare: AI agents can assist in diagnostics, treatment planning, and patient care. IBM Watson AI uses AI agents to analyze patient data and suggest personalized treatment plans.
AI agents are progressing and making their mark in fields like:
- AI-enabled customer experience (CX): They are used for personalized recommendations and interactive chatbots. AI-powered chatbots like Drift engage customers in customized conversations for better CX.
- Generative AI and AI-assisted decision-making: AI agents play a role in the creation of new content in media and entertainment with the support for complex analysis and strategic planning. Innovations like Humane AI Pin and Rabbit AI-powered R1 are great examples of the future
Now, let’s discuss the tools we will be using for the tutorial.
Introducing Anthropic's Claude
Claude background
Claude is a generative pre-trained transformer developed by Anthropic, first released in March 2023. Initially designed to predict the next word in large text datasets, Claude was fine-tuned with Constitutional AI to ensure it is helpful, honest, and harmless. The Claude family has evolved through several versions, with each iteration expanding its capabilities and context window, culminating in the highly advanced Claude 3 series.
Claude capabilities
- Large context handling: can process up to 200,000 tokens, with some versions reaching 1 million tokens
- Document processing: upload and analyze PDFs and other documents for summarization and task assistance
- Coding and workflow: enhanced capabilities in coding, multistep workflows, and chart interpretation
- Real-time code preview: create and preview code, such as websites or SVGs, within the interface
- Meta-cognitive reasoning: ability to recognize artificial testing scenarios, improving accuracy and reliability
Claude can automate workflows by converting natural language into structured API calls and orchestrating subagents for complex tasks, such as optimizing meeting schedules based on availability. Out of many solutions, why should you use Claude as an AI agent? Here’s why:
- Tool integration: seamlessly interacts with external tools and APIs for efficient task automation
- Accurate data handling: extracts structured data from unstructured text, reducing manual work
- Dynamic responses: provides precise, real-time answers by searching databases or using web APIs
- Workflow automation: converts natural language requests into structured API calls for streamlined operations
What is pgai?
Pgai is a PostgreSQL extension that simplifies building AI-powered applications directly within the database. It brings AI models closer to data, enabling efficient in-database execution of tasks like vector embedding, content generation, and data reasoning.
Key features
- It creates vector embeddings within SQL queries.
- It integrates with LLMs, retrieving chat completions and generating content using models like Claude, OpenAI, and Cohere.
- It enables data reasoning so you can classify, summarize, and enrich data directly in PostgreSQL.
Pgai seamlessly integrates with PostgreSQL, enabling reduced latency, a simplified architecture, and enhanced AI capabilities directly within the database environment. Let’s put these concepts together to build our own AI agent.
Teaching Claude to Use Tools With pgai
In this section, we will outline a step-by-step guide about using pgai with Claude to build a custom AI weather assistant designed to handle weather queries.
Set up
To build an AI agent that can handle real-time weather queries, you’ll need to set up PostgreSQL with the pgai extension and configure it to use Claude models. Here’s how to get started:
Setting up PostgreSQL
We'll use Docker to quickly set up PostgreSQL with TimescaleDB. Follow these steps:
Prerequisites:
- Docker
psql
(PostgreSQL command-line tool)
Install and configure PostgreSQL with TimescaleDB
1. Pull the TimescaleDB Docker image
Use the following command to pull the TimescaleDB image:
docker pull timescale/timescaledb-ha:pg16
The TimescaleDB extension is pre-installed in the default postgres
database in this Docker image. It will be available in any new database you create.
2. Run the Docker container
Start the Docker container with this command:
docker run -d --name timescaledb -p 5432:5432 -e POSTGRES_PASSWORD=password timescale/timescaledb-ha:pg16
The --network host
option allows the container to use the host’s network stack, which facilitates communication with other services. If you’re running multiple instances, make sure to use different ports for each.
3. Connect to your PostgreSQL instance
To connect to your PostgreSQL database, use the psql
command:
psql -d "postgres://postgres:password@localhost:5432/postgres"
If psql
is not installed, follow the installation guide for your system. The default user and database for the timescaledb-ha:pg16
image are both postgres
, and this command should establish a successful connection if the setup is correct.
Integrate PostgreSQL with pgai
1. Ensure the pgai extension is installed
Ensure the pgai
extension is installed and properly configured in your PostgreSQL setup. This extension allows you to use AI models directly within PostgreSQL. After connecting to your PostgreSQL database, you can check the installed extensions using the following command:
\dx
2. Installing pgai: If the ai extension is not listed, install it with this query:
CREATE EXTENSION IF NOT EXISTS ai CASCADE;
The CASCADE
option ensures that the pgvector
and plpython3u
extensions are installed automatically if they are not already present. Once the installation is complete, you can exit the database connection.
3. Required libraries
To communicate with PostgreSQL from Python, you will need the psycopg2
library. Since Docker images for PostgreSQL are pre-packaged with Python and Pip, you can easily install psycopg2
with the following command:
pip install psycopg2
OpenWeather API
- Get an API key: create an account on the OpenWeatherMap.
- Generate key: go to the API keys page and copy your API key.
- Choose API endpoint: pick an endpoint from the API documentation (e.g., Current Weather Data).
These steps will set up pgai
and the necessary Python libraries to integrate with PostgreSQL and build your AI agent.
Implementation
A tool is a module or function that can be executed. When a query is presented to the LLM, it interprets the query and selects which tool to call from a list based on the query's meaning. Let’s build our weather tool.
Imports
The following imports are required in Python to get started with the tutorial:
import psycopg2
import requests
Tools
To get the weather for a city using the API, we first need to convert the city name into latitude and longitude. Therefore, we create a get_coordinates
function to obtain these coordinates, which is then called by the get_weather
function to retrieve the weather information.
1. get_coordinates
Function:
- Purpose: it retrieves the latitude and longitude of a city.
- Parameters:
api_key
,city
, optionalstate
,country
. - Process:
- Constructs URL with city, state, and country
- Sends a GET request to OpenWeatherMap's geocoding API
- Parses response to extract latitude and longitude or raises an error if the request fails
def get_coordinates(api_key, city, state=None, country=None):
"""
Fetches the latitude and longitude for a given city using OpenWeatherMap's geocoding API.
:param api_key: Your API key for OpenWeatherMap.
:param city: The name of the city.
:param state: Optional state code.
:param country: Optional country code.
:return: A tuple containing latitude and longitude.
"""
base_url = "http://api.openweathermap.org/geo/1.0/direct"
params = {
'q': f"{city},{state},{country}" if state and country else f"{city},{state}" if state else f"{city}",
'limit': 1,
'appid': api_key
}
response = requests.get(base_url, params=params)
data = response.json()
if response.status_code == 200 and data:
lat = data[0]['lat']
lon = data[0]['lon']
return lat, lon
else:
raise Exception("Failed to get coordinates")
2. get_weather
Function:
- Purpose: fetches current weather for a city using coordinates
- Parameters:
api_key
,city
, optionalstate
,country
. - Process:
- Calls
get_coordinates
to get latitude and longitude - Uses these coordinates to request weather data from OpenWeatherMap’s weather API
- Parses and returns weather details or an error message
- Calls
def get_weather(api_key, city, state=None, country=None):
"""
Fetches the current weather for a given city.
:param api_key: Your API key for OpenWeatherMap.
:param city: The name of the city.
:param state: Optional state code.
:param country: Optional country code.
:return: A dictionary with weather information.
"""
try:
lat, lon = get_coordinates(api_key, city, state, country)
except Exception as e:
return {'error': str(e)}
weather_base_url = "https://api.openweathermap.org/data/2.5/weather"
weather_params = {
'lat': lat,
'lon': lon,
'appid': api_key,
'units': 'metric' # Use 'imperial' for Fahrenheit
}
response = requests.get(weather_base_url, params=weather_params)
weather_data = response.json()
if response.status_code == 200:
weather = {
'city': weather_data['name'],
'temperature': weather_data['main']['temp'],
'description': weather_data['weather'][0]['description'],
'humidity': weather_data['main']['humidity'],
'pressure': weather_data['main']['pressure']
}
else:
weather = {
'error': weather_data.get('message', 'Failed to retrieve data')
}
return weather
After calling the function for the city of London, we receive the following results:
{'city': 'London', 'temperature': 19.88, 'description': 'overcast clouds', 'humidity': 57, 'pressure': 1016}
The tools are ready; let’s integrate them with pgai.
AI agent with Pgai
We will create a Python function that will connect to the PostgreSQL client and provide the tool that needs to be used, given the query. Let’s see the code, and then we will explain it afterward:
import psycopg2
def choose_tool(api_key, query):
"""
Executes a query on the PostgreSQL database to select and use a tool based on the provided content.
This function interacts with the PostgreSQL database using the pgai extension to generate a response
by calling the `public.anthropic_generate` function. The tool named 'get_weather' is specified to
provide weather information for a given location based on the user content.
:param api_key: Your API key for the AI service (used in the query).
:param content: The content of the user message that specifies the task or query.
:return: The result of the query execution from the AI function.
"""
# Connection parameters
connection_string = "postgres://postgres:password@localhost:5432/postgres"
query = """
SELECT anthropic_generate(
_model => 'claude-3-5-sonnet-20240620',
_messages => jsonb_build_array(
jsonb_build_object(
'role', 'user',
'content', %s
)
),
_max_tokens => 1024,
_api_key => %s,
_temperature => 0.7,
_tools => jsonb_build_array(
jsonb_build_object(
'name', 'get_weather',
'description', 'Get the current weather in a given location',
'input_schema', jsonb_build_object(
'type', 'object',
'properties', jsonb_build_object(
'location', jsonb_build_object(
'type', 'string',
'description', 'The city and state, e.g. San Francisco, CA'
)
),
'required', jsonb_build_array('location')
)
)
)::jsonb
);
"""
try:
# Create a connection object
conn = psycopg2.connect(connection_string)
# Create a cursor object
cursor = conn.cursor()
# Execute the query with content and API key
cursor.execute(query, (content, api_key))
# Fetch the result
result = cursor.fetchone()
return result
except Exception as e:
print("Error executing query:", e)
return None
finally:
# Close the cursor and connection
if cursor:
cursor.close()
if conn:
conn.close()
The main part of the function above is the query made to the database. The anthropic_generate
function uses several parameters to generate a response. The _model
parameter specifies the AI model as claude-3-5-sonnet-20240620
. The _messages parameter defines the input messages as a JSONB
array, with the message content provided dynamically. The _max_tokens
parameter limits the response to 1,024 tokens, while the _api_key
parameter uses the provided API key. The _temperature
parameter sets the creativity level of the response to 0.7.
The _tools
parameter provides a list of tools in JSONB
format, including the tool name get_weather
, a description of its purpose, and an input_schema
that defines it as an object with properties such as location
, which is a required field.
Calling the function with the following query gives us the following result:
query = "What's the weather like in San Francisco?"
response = generate_response(api_key, query)
The content here is the main part. Given the query, Claude detects which tool to use and the input that will go into the function.
content=[TextBlock(text="Certainly! I can help you get the current weather information for San Francisco. To do that, I'll use the get_weather function. Let me fetch that information for you right away.", type='text'), ToolUseBlock(id='toolu_01G1YeYM3wHpUDxKXgq92wsj', input={'location': 'San Francisco'}, name='get_weather', type='tool_use')]
Now, with some control flow, we can call the function with the required argument.
tool_use_block = response.content[1]
if tool_use_block.name == "get_weather":
print(get_weather(api_key, tool_use_block.input['location']))
Testing and validation
We are done with the implementation. After testing and validating, the results are as follows:
{'city': 'San Francisco', 'temperature': 13.38, 'description': 'overcast clouds', 'humidity': 91, 'pressure': 1014}
Here we have it. Our own AI weather assistant is ready. Just give it a query in natural language, and it will provide the output.
Here are some best practices for implementing AI agents with tools like pgai:
1. Token usage monitoring: Regularly monitor the usage attribute in your responses to keep track of input and output tokens. This helps manage costs effectively and optimize the usage of API calls. Here’s how to use it:
response.usage
Here’s what it looks like:
Usage(input_tokens=353, output_tokens=93)
2. Tool selection and validation: Ensure that tools provided to the AI agent are clearly defined with accurate input schemas. Validate the inputs before executing the tools to prevent errors and ensure the agent functions as expected.
3. Graceful error handling: Implement robust error handling for database queries and API calls. If a tool fails or returns an unexpected response, your AI agent should handle the situation by providing informative feedback or fallbacks instead of causing service disruptions.
4. Security and API key management: Securely store and manage API keys and other sensitive information. Use environment variables or secure vaults to store API keys and implement access controls to prevent unauthorized access.
Benefits of Pgai With Claude
We've explored the implementation details with pgai and Claude. We didn’t combine them by chance; they offer specific benefits when used together:
- Enhanced efficiency: Integrating pgai with Claude enhances efficiency by allowing AI models to be executed directly within the database, reducing latency and simplifying the workflow. This tight integration minimizes data movement, leading to faster query execution and better response times.
- Scalability: The combination of pgai with Claude scales seamlessly, enabling the handling of larger datasets and more complex queries as demand grows.
- Cost-effectiveness: Utilizing in-database AI agents reduces operational costs by eliminating the need for external API calls and minimizing data transfer. By keeping computations within the database, you reduce reliance on external resources, leading to significant cost savings over time. Conversely, Claude Sonnet 3.5 is priced at just a fifth of its predecessors while also delivering superior performance. This combination makes pgai and Claude an ideal pairing.

Conclusion
AI agents represent the next significant advancement following large language models. Unlike LLMs, these agents not only comprehend language but are also capable of taking appropriate actions based on that understanding. In this post, we discuss the main idea behind AI agents, their impact on the various industries, and implemented one with pgai and Claude.
Using Claude with pgai allows you to create AI agents directly within the database, offering benefits like efficiency, scalability, and cost-effectiveness. With pgai, implementing AI agents becomes so simple that anyone can create one.
Start using pgai to streamline your AI pipelines and integrate AI directly within your database, eliminating the need for additional libraries. Pgai is open source under the PostgreSQL License and is available for you to use in your AI projects today. You can find installation instructions on the pgai GitHub repository (GitHub ⭐s welcome!). You can also access pgai on any database service on Timescale’s cloud PostgreSQL platform.